- v7.0 版本: 新增实例分割,现在可以训练、测试、检测、导出实例分割模型,目前视觉领域的三大任务目标检测、分类、分割都可以用yolov5工程了
- 支持飞桨PaddlePaddle的导出和推理了
- v6.2 版本: 新增yolov5分类模型, 适配苹果M1/M2芯片,引入ClearML和Deci.ai。
- v6.1 版本: 现在支持11种格式的模型导出和推理,更新了预训练权重,指标上相较v6.0好了一点。
现在支持导出和推理的模型有:PyTorch(.pt)
,TorchScript(.torchscript)
,ONNX(.onnx)
,OpenVINO
,TensorRT(.engine)
,CoreML(.mlmodel)
,TensorFlow SavedModel
,Tensorflow GraphDef(.pb)
,TensorFlow Lite(.tflite)
,TensorFlow Edge TPU(.tflite)
,Tensorflow.js
。 - v6.0 版本: 新增yolov5 nano模型,其他模型结构也有修改,模型转换/导出友好,精度基本不变,速度略有提升。
本仓库Fork自Ultralytics公司出品的yolov5,原仓库地址为:ultralytics/yolov5 ,版权请参见原仓库License
点击展开
yolov5按从小到大分为五个模型yolov5n、yolov5s、yolov5m、yolov5l、yolov5x,这五个模型的表现见下图:
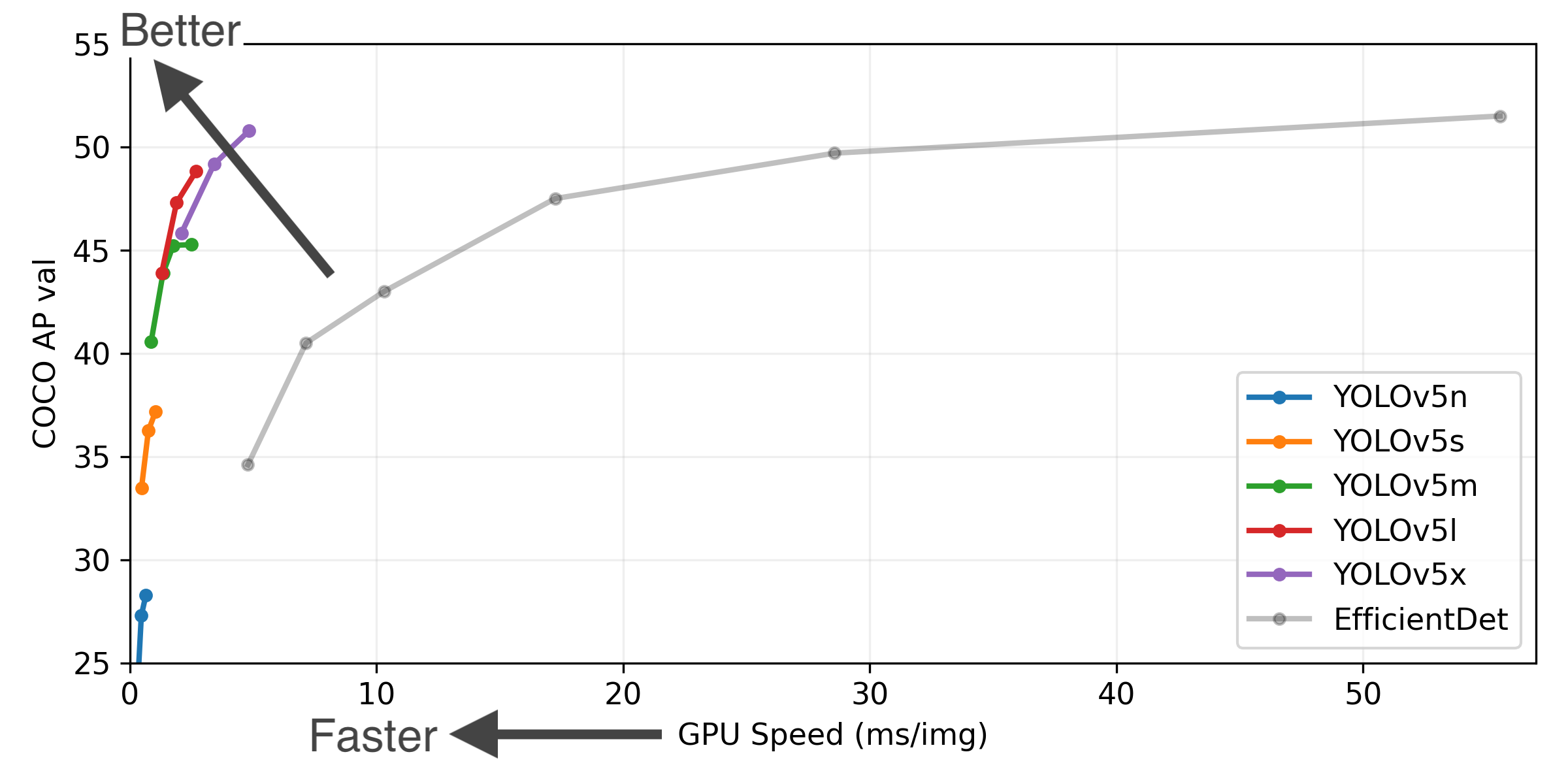
在这五个模型基础上增加P6层,模型结构更深,四个模型效果分别为:
- 2022年8月17日:v6.2 release
- 2022年2月22日:v6.1 release
- 2021年10月12日:v6.0 release
- 2021年4月12日:v5.0 release
- 2021年1月5日:v4.0 release
- 2020年10月29日:v3.1 release
- 2020年8月13日: v3.0 release
- 2020年7月23日: v2.0 release
- 2020年6月26日: v1.0 release
注意:yolov5更新很快,永远建议使用最新版本代码,我不能及时更新的话请去官方repo下更新。
Python版本需要 ≥ 3.6.0,使用GPU的话要有CUDA环境。其他依赖都写在了requirements.txt 里面。一键安装依赖的话,打开命令行,cd到yolov5的文件夹里,输入:
$ pip install -r requirements.txt
有安装多个版本python的,记得在命令行里把开头的pip
替换为pip3
,python
也换为你的版本,如python3
或者python3.8
,下同。
pip安装慢的,请配置镜像源,下面是清华的镜像源。
$ pip install pip -U
$ pip config set global.index-url https://pypi.tuna.tsinghua.edu.cn/simple
想配其他镜像源直接把网址替换即可,以下是国内常用的镜像源:
豆瓣 https://pypi.doubanio.com/simple/
网易 https://mirrors.163.com/pypi/simple/
阿里云 https://mirrors.aliyun.com/pypi/simple/
腾讯云 https://mirrors.cloud.tencent.com/pypi/simple
清华大学 https://pypi.tuna.tsinghua.edu.cn/simple/
注意事项
- Windows版在检测(
detect.py
)时可能有问题,请尽量在Linux或者MacOS使用本工程。如果你是CUDA11,且检测不出框,则降级到CUDA10.2,然后重装Pytorch1.8.1+cu102试试。 - 不想自己配环境的可以用colab或者docker,下面是几个免费环境:
- Google Colab and Kaggle :
- Google Cloud : GCP 快速上手教程
- Amazon Deep Learning AMI. See AWS 快速上手教程
- Docker Image. Docker 快速上手教程
直接执行下面命令。根据你的显卡情况,使用最大的 --batch-size
,(下列命令中的batch size是16G显存的显卡推荐值)。其中,--cfg
为指定模型结构,--weights
为指定初始化模型权重。
$ python train.py --data coco.yaml --cfg yolov5s.yaml --weights '' --batch-size 64
yolov5m.yaml 40
yolov5l.yaml 24
yolov5x.yaml 16
使用自己的训练集进行自定义训练,详情点击展开:
① 准备标签
yolo格式的标签为txt格式的文件,文件名跟对应的图片名一样,除了后缀改为了.txt。 具体格式如下:
- 每个目标一行,整个图片没有目标的话不需要有txt文件,或者空文件。
- 每行的格式为
class_num x_center y_center width height
- 其中
class_num
取值为0
至total_class - 1
,框的四个值x_center
y_center
width
height
是相对于图片分辨率大小正则化的0-1
之间的数,左上角为(0,0)
,右下角为(1,1)
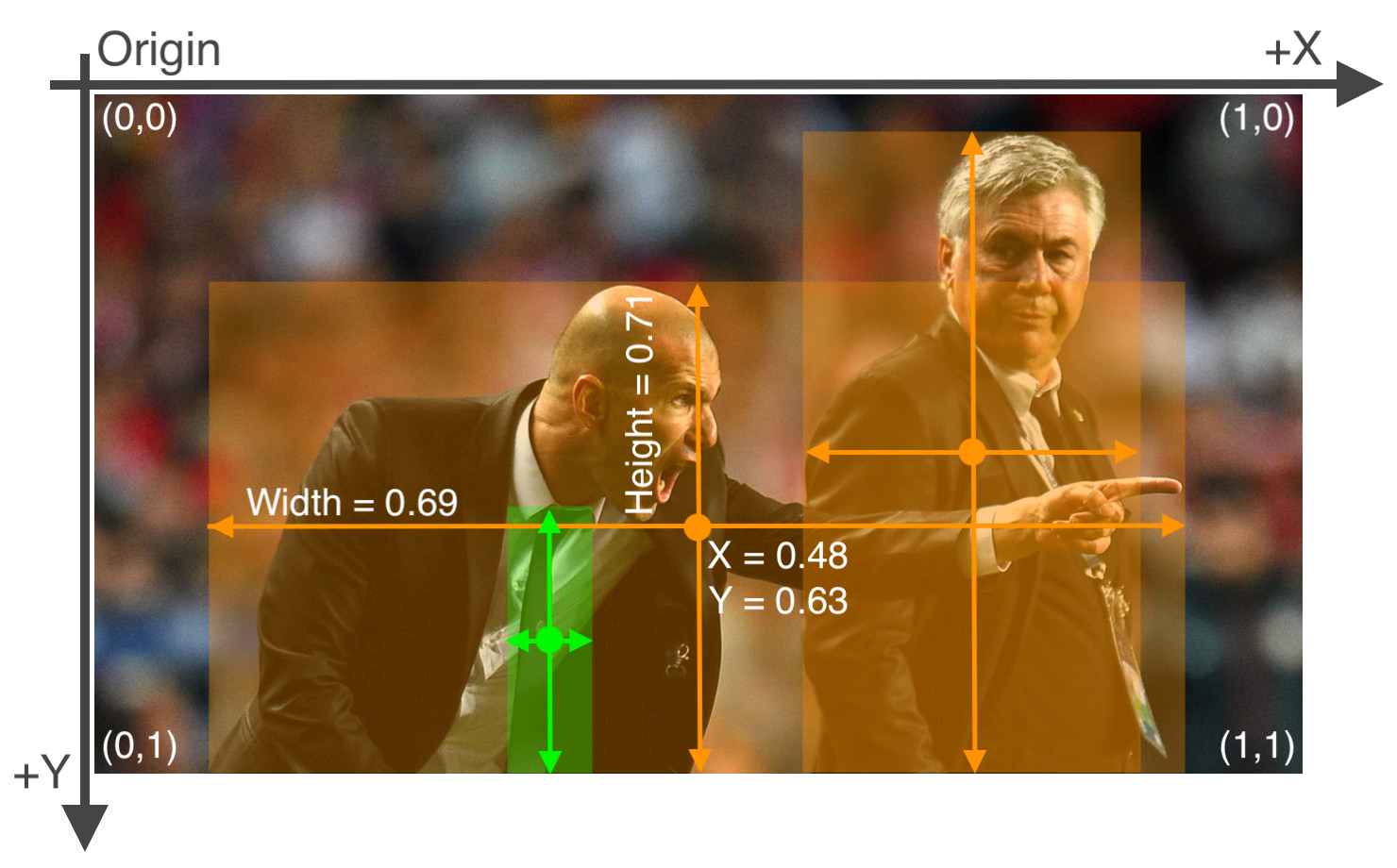
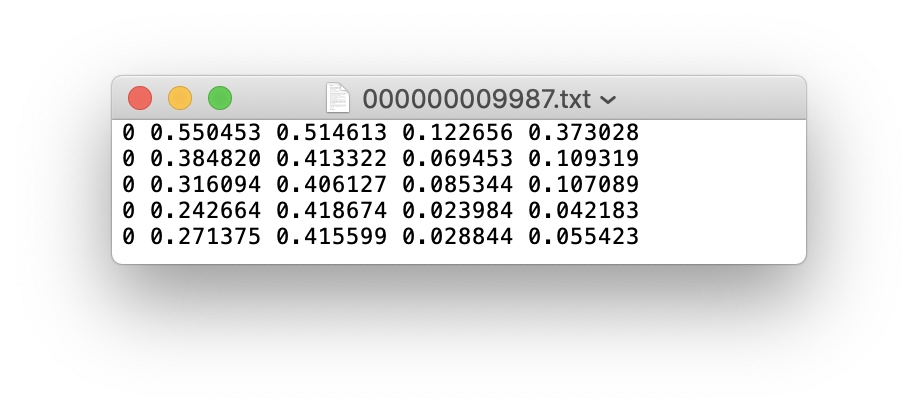
② 数据存放规范
数据读取有三种形式,但是还是推荐下面这个存储方式。因为yolov5只需要给图片路径即可,代码会根据图片找标签,具体形式的把图片路径/images/*.jpg
替换为/labels/*.txt
,所以要新建两个文件夹,一个名为images
存放图片,一个名为labels
存放标签txt文件,如分训练集、验证集和测试集的话,还要再新建各自的文件夹,如图:
③ 准备yaml文件
自定义训练需要修改.yaml文件,一个是模型文件(可选),一个是数据文件。
- 模型文件(可选):可以根据你选择训练的模型,根据需要修改
./models
里的yolov5s.yaml
/yolov5m.yaml
/yolov5l.yaml
/yolov5x.yaml
文件,
注意 :当需要随机初始化或者修改模型结构时才会使用本文件,如未修改模型结构,推荐使用预训练权重初始化,
如python3 train.py --weights yolov5s.pt
,
而不是python3 train.py --weights '' --cfg yolov5s.yaml
- 数据文件:根据
./data
文件夹里的coco数据文件,制作自己的数据文件,在数据文件中定义训练集、验证集、测试集路径;定义总类别数;定义类别名称# train and val data as 1) directory: path/images/, 2) file: path/images.txt, or 3) list: [path1/images/, path2/images/] train: ../coco128/images/train2017/ val: ../coco128/images/val2017/ test:../coco128/images/test2017/ # number of classes nc: 80 # class names names: ['person', 'bicycle', 'car', 'motorcycle', 'airplane', 'bus', 'train', 'truck', 'boat', 'traffic light', 'fire hydrant', 'stop sign', 'parking meter', 'bench', 'bird', 'cat', 'dog', 'horse', 'sheep', 'cow', 'elephant', 'bear', 'zebra', 'giraffe', 'backpack', 'umbrella', 'handbag', 'tie', 'suitcase', 'frisbee', 'skis', 'snowboard', 'sports ball', 'kite', 'baseball bat', 'baseball glove', 'skateboard', 'surfboard', 'tennis racket', 'bottle', 'wine glass', 'cup', 'fork', 'knife', 'spoon', 'bowl', 'banana', 'apple', 'sandwich', 'orange', 'broccoli', 'carrot', 'hot dog', 'pizza', 'donut', 'cake', 'chair', 'couch', 'potted plant', 'bed', 'dining table', 'toilet', 'tv', 'laptop', 'mouse', 'remote', 'keyboard', 'cell phone', 'microwave', 'oven', 'toaster', 'sink', 'refrigerator', 'book', 'clock', 'vase', 'scissors', 'teddy bear', 'hair drier', 'toothbrush']
④ 进行训练
训练直接运行train.py
即可,后面根据需要加上指令参数,--weights
指定权重,--data
指定数据文件,--batch-size
指定batch大小,--epochs
指定epoch。一个简单的训练语句:
# 使用yolov5s模型训练coco128数据集5个epochs,batch size设为16
$ python train.py --batch 16 --epochs 5 --data ./data/coco128.yaml --weights ./weights/yolov5s.pt
各个训练指令的详细说明,新手的话只需要关注这几个加⭐的指令,点击展开:
有参指令(后面要加相应参数)
--weights
(⭐)指定模型权重,如果不加此参数会默认使用COCO预训的yolov5s.pt
作为初始化权重,--weights ''
则会随机初始化权重--cfg
指定模型文件,当使用--weights ''
时,需用此命令指定模型文件。--data
(⭐)指定数据文件--hyp
指定超参数文件--epochs
(⭐)指定epoch数,默认300--batch-size
--batch
(⭐)指定batch大小,默认16
,传-1
的话就是autobatch--img-size
--img
--imgsz
(⭐)指定训练图片大小,默认640
--cache
缓存到ram
或者disk
以加速训练--device
(⭐)指定训练设备,如--device 0,1,2,3
--local_rank
分布式训练参数,不要自己修改!--workers
指定dataloader的workers数量,默认8
--project
训练结果存放目录,默认./runs/train/--name
训练结果存放名,默认exp--label-smoothing
标签平滑处理,默认0.0
--bbox_interval
设置W&B的标签图片显示间隔?没用过--save_period
设置多少个epoch保存一次模型--artifact_alias
W&B用哪个版本的数据集--freeze
冻结模型层数,默认0不冻结,冻结主干网就传10,冻结所有就传24--patience
多少个epoch没有提升就终止训练,默认100
无参指令
--rect
矩形训练--resume
继续训练,默认从最后一次训练继续--nosave
训练中途不存储模型,只存最后一个checkpoint--noval
训练中途不在验证集上测试,训练完毕再测试--noautoanchor
关闭自动锚点检测--evolve
超参数演变--bucket
使用谷歌gsutil bucket--image-weights
训练中对图片加权重--multi-scale
训练图片大小+/-50%变换--single-cls
单类训练--adam
使用torch.optim.Adam()优化器--sync-bn
使用SyncBatchNorm,只在分布式训练可用--entity
W&B的参数,不懂--exist-ok
如训练结果存放路径重名,不覆盖已存在的文件夹--quad
使用四合一dataloader,详见原作者说明--linear-lr
线性学习率--upload_dataset
上传数据集至W&B
推理支持多种模式,图片、视频、文件夹、rtsp视频流和流媒体都支持。
直接执行detect.py
,指定一下要推理的目录即可,如果没有指定权重,会自动下载默认COCO预训练权重模型。手动下载:v6.0权重阿里云盘下载地址 (将后缀改为zip解压即可)。
推理结果默认会保存到 ./runs/detect
中。
# 快速推理,--source 指定检测源,以下任意一种类型都支持:
$ python detect.py --source 0 # 本机默认摄像头
image.jpg # 图片
video.mp4 # 视频
path/ # 文件夹下所有媒体
path/*.jpg # 使用glob模块匹配文件
screen # 检测本机屏幕
rtsp://170.93.143.139/rtplive/470011e600ef003a004ee33696235daa # rtsp视频流
http://112.50.243.8/PLTV/88888888/224/3221225900/1.m3u8 # http视频流
使用权重./weights/yolov5s.pt
去推理./data/images
文件夹下的所有媒体,并且推理置信度阈值设为0.5:
$ python detect.py --source ./data/images/ --weights ./weights/yolov5s.pt --conf 0.5
自己根据需要加各种指令,新手只需关注带⭐的指令即可。
有参
--source
(⭐)指定检测来源,详见上面的介绍--weights
(⭐)指定权重,不指定的话会使用yolov5s.pt预训练权重--img-size
--imgsz
--img
(⭐)指定推理图片分辨率,默认640,三个指令一样--conf-thres
(⭐)指定置信度阈值,默认0.4,也可使用--conf
--iou-thres
指定NMS(非极大值抑制)的IOU阈值,默认0.5--max-det
每张图最多检测多少目标--device
指定设备,如--device 0
--device 0,1,2,3
--device cpu
--classes
只检测特定的类,如--classes 0 2 4 6 8
--project
指定结果存放路径,默认./runs/detect/--name
指定结果存放名,默认exp--line-thickness
画图时线条宽度
无参
--view-img
图片形式显示结果--save-txt
输出标签结果(yolo格式)--save-conf
在输出标签结果txt中同样写入每个目标的置信度--save-crop
从图片\视频上把检测到的目标抠出来保存--nosave
不保存图片/视频--agnostic-nms
使用agnostic NMS(前背景)--augment
增强识别,速度会慢不少。详情--visualize
特征可视化--update
更新所有模型--exist-ok
若重名不覆盖--hide-labels
隐藏标签--hide-conf
隐藏置信度--half
半精度检测(FP16)--dnn
在onnx推理中使用OpenCV DNN
首先明确,推理是直接检测图片,而测试是需要图片有相应的真实标签的,相当于检测图片后再把推理标签和真实标签做mAP计算。
例如使用./weights/yolov5x.pt
权重检测./data/coco.yaml
里定义的测试集,图片分辨率设为672。
$ python val.py --weights ./weights/yolov5x.pt --data ./data/coco.yaml --img 672
有参
--weights
(⭐)测试所用权重,默认yolov5sCOCO预训练权重模型--data
(⭐)测试所用的.yaml文件,默认使用./data/coco128.yaml
--batch-size
测试用的batch大小,默认32,这个大小对结果无影响--img-size
--imgsz
--img
测试集分辨率大小,默认640,测试建议使用训练分辨率--conf-thres
目标置信度阈值,默认0.001--iou-thres
NMS的IOU阈值,默认0.65--task
指定任务模式,train, val, test, speed或者study--device
指定设备,如--device 0
--device 0,1,2,3
--device cpu
--project
指定结果存放路径,默认./runs/test/--name
指定结果存放名,默认exp
无参
--single-cls
所有类别视为一类--augment
增强识别--verbose
输出各个类别的mAP--save-txt
输出标签结果(yolo格式)--save-hybrid
输出标签+预测混合结果到txt--save-conf
保存置信度至输出文件中--save-json
保存结果为json--exist-ok
若重名不覆盖--half
半精度检测(FP16)
- 翻译官方Tutorial的各个教程
- 建一个交流群
如有疑问可在本repo的Issues里提,我看到会及时回复。
如有代码bug请去yolov5官方Issues下提。
个人联系方式:[email protected]
遵循yolov5官方LICENSE
本README所有内容禁止除fork外的任何形式的转载