Hi,
Thank you for sharing your code on GitHub and congratulations on your TGRS 2020 paper (it's a great piece of work). I have two questions about the training phase of the DDCM model. I would really appreciate it if you could help me find their answers:
1- Can anyone please tell me when the training process ends in train.py
? I have read both the paper and code and have not been able to find a hint on this matter. I am referring specifically to training on the Vaihingen dataset. There appears to be a continuous reduction in the lr
, but I am unsure when this reduction is stopped since there is no maximum number of epoch
s, minimum lr
, etc. defined in the configurations. There is only one restriction defined: the maximum number of iterations is 10e8
. Does this mean that the training should continue for 10e8
iterations? Since each iteration lasts for 1 minute on my computer (batch size =5
), 10e8
iterations will take forever!
2- I ran your code with the Vaihingen dataset for approximately 16 epochs (16k iterations) and the following are the training and validation loss trends.
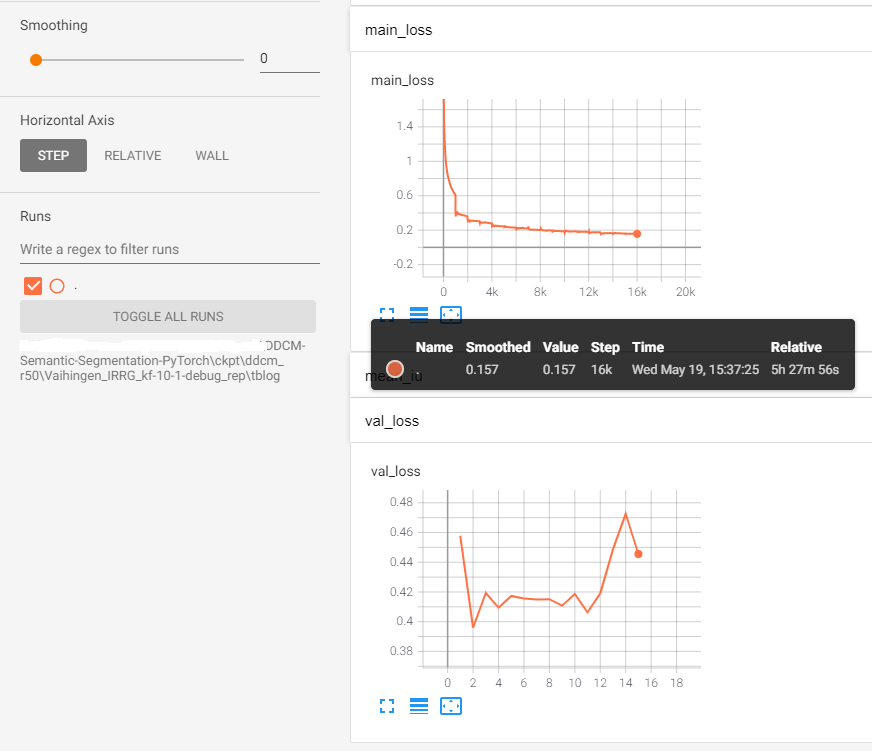
As can be seen in the figure, the main_loss
is reduced (with a few abrupt steps) from 1.5 to 0.157 (black box in the middle of the figure belongs to main_loss
at 16k iteration). However, the val_loss
keeps fluctuating between 0.40 and 0.47 instead of decreasing, e.g., in epoch=16
, main_loss
equals 0.157
, while val_loss
equals 0.47
. So, according to the figure, training loss decreases during training, but validation loss does not. This means that we are dealing with a large gap between the train and validation losses (overfitting). Have you observed this behavior in your trainings? Do you have any specific suggestions, solutions, or comments to fix the overfitting problem?