去噪扩散模型,根据论文 Denoising Diffusion Probabilistic Models (DDPM) 实现, ddpm_conditional.py
是根据类别进行训练和生成图像。 ddpm_conditional.py
代码实现了Classifier-Free-Guidance (CFG) & Exponential-Moving-Average (EMA)。
参考代码链接:
https://github.com/dome272/Diffusion-Models-pytorch
https://github.com/tcapelle/Diffusion-Models-pytorch
参考视频YouTube链接:
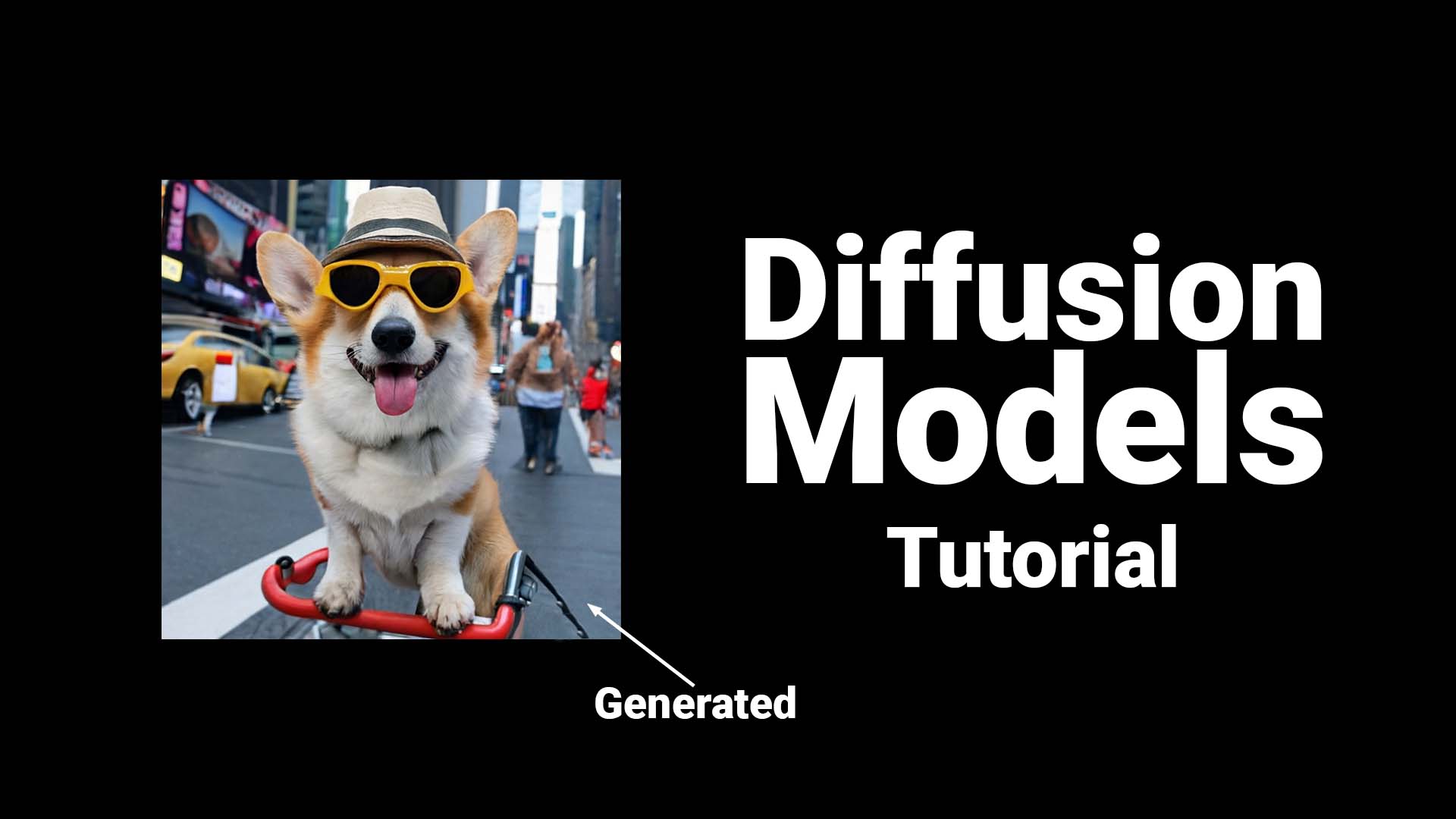
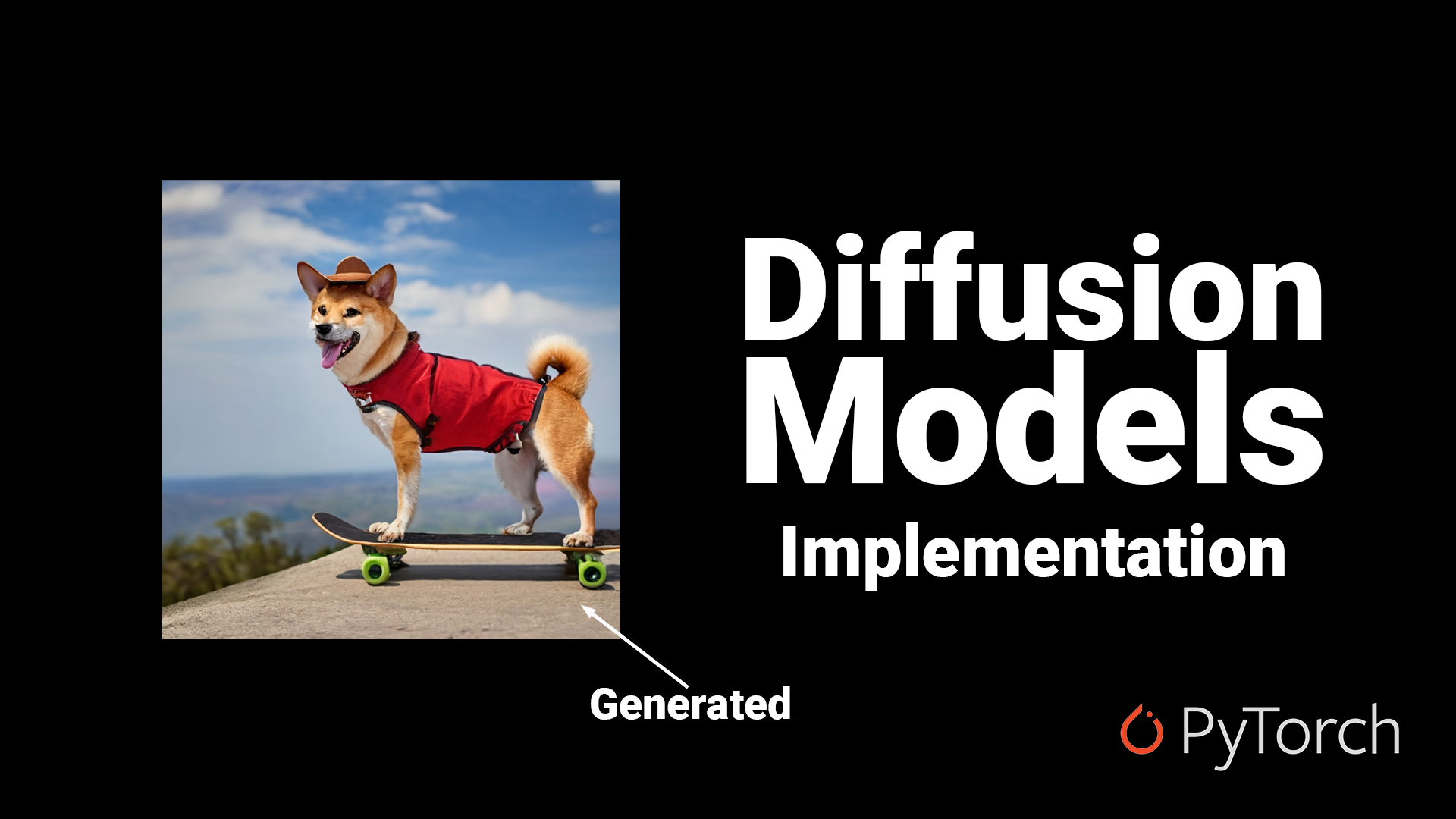
参考视频B站链接:
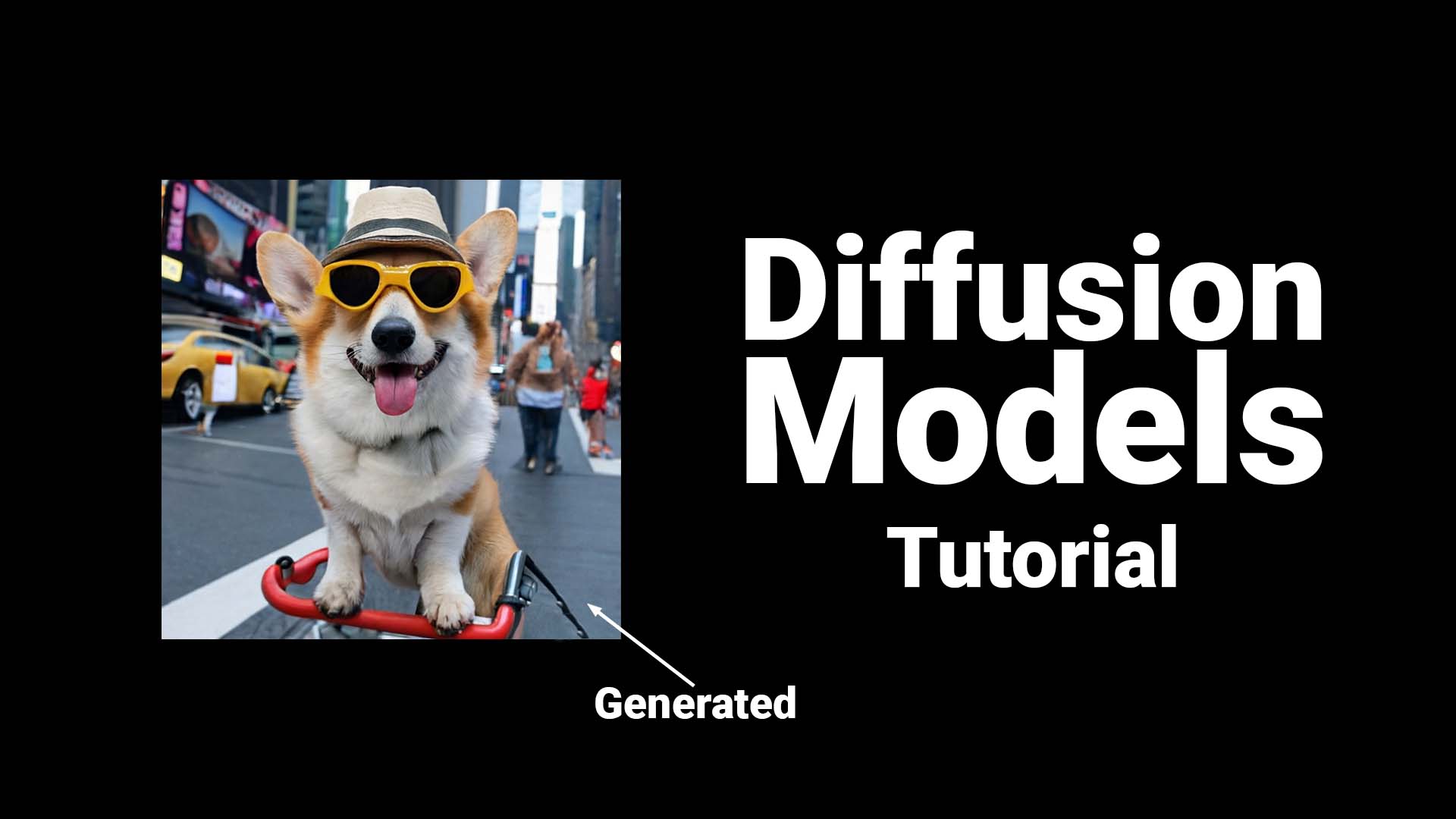
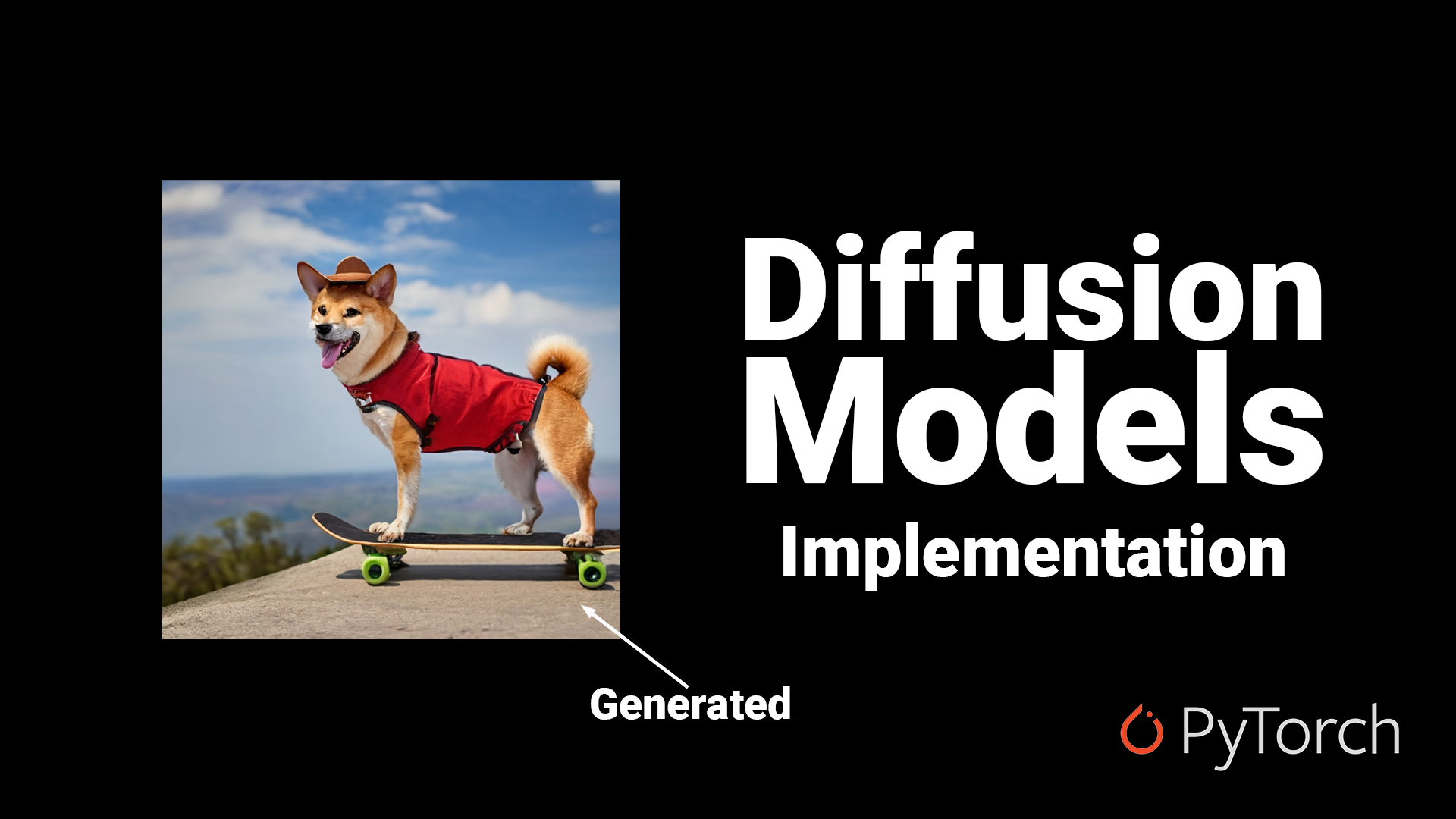
.
│ ddpm_conditional.py # 1.扩散模型主程序,实现了DDPM类,和训练代码
│ modules.py # 2.网络模块,实现了Unet和Unet_conditional
│ utils.py # 3.一些辅助工具
│ README.md # 说明
│
├─imgs # 存放一些图片
│
├─models # 模型权重文件夹
│ └─DDPM_conditional
│
├─results # 训练过程中采样图文件夹
│ └─DDPM_conditional
│
└─runs # 运行日志文件夹
└─DDPM_conditional
CIFAR-10 64x64, 10 classes
airplane:0,
auto:1,
bird:2,
cat:3,
deer:4,
dog:5,
frog:6,
horse:7,
ship:8,
truck:9
python ddpm_conditional.py
n = 10
device = "cuda"
model = UNet_conditional(num_classes=10).to(device)
ckpt = torch.load("conditional_ema_ckpt.pt")
model.load_state_dict(ckpt)
diffusion = Diffusion(img_size=64, device=device)
y = torch.Tensor([6] * n).long().to(device)
x = diffusion.sample(model, n, y, cfg_scale=3)
plot_images(x)
训练 | 采样 |
---|---|
![]() |
![]() |
展开/折叠
class Diffusion:
def __init__(self, noise_steps=1000, beta_start=1e-4, beta_end=0.02, img_size=256, device="cuda"):
self.noise_steps = noise_steps
self.beta_start = beta_start
self.beta_end = beta_end
self.img_size = img_size
self.device = device
self.beta = self.prepare_noise_schedule().to(device)
self.alpha = 1. - self.beta
self.alpha_hat = torch.cumprod(self.alpha, dim=0)
def prepare_noise_schedule(self):
'''
Generate noise beta of each time step
return: shape (noise_steps, )
'''
return torch.linspace(self.beta_start, self.beta_end, self.noise_steps)
def sample_timesteps(self, n):
'''
Sample time steps for each image
input:
n: batch_size, int
return:
t: time_step, shape (n, ), values in [1, noise_step]
'''
return torch.randint(low=1, high=self.noise_steps, size=(n, ))
def noise_images(self, x, t):
'''
Add noise process: x_0 -> x_{t}
input:
x: input_images, shape (batch_size, 1, img_size, img_size)
t: time_step, int
return:
noise_images: shape (batch_size, 1, img_size, img_size)
noise: shape (batch_size, 1, img_size, img_size)
noise_images = sqrt(alpha_hat[t]) * x + sqrt(1 - alpha_hat[t]) * noise
'''
sqrt_alpha_hat = torch.sqrt(self.alpha_hat[t])[:, None, None, None]
sqrt_one_minus_alpha_hat = torch.sqrt(1 - self.alpha_hat[t])[:, None, None, None]
Ɛ = torch.randn_like(x)
return sqrt_alpha_hat * x + sqrt_one_minus_alpha_hat * Ɛ, Ɛ
def sample(self, model, n, labels, cfg_scale=3):
'''
Denoise process: x_{t} -> x_{t-1} -> ... -> x_0
input:
model: nn.Module
n: batch_size, int
labels: shape (n, ), values in [0, 9]
cfg_scale: float, 0.0 ~ 1.0, 0.0: unconditioned diffusion, 1.0: conditioned diffusion
return:
x_0: images in t0, shape (n, 1, img_size, img_size), values in [0, 255]
sampled_images (x_{t-1}) = 1 / sqrt(alpha[t]) * (noisy_images (x_t) - (1 - alpha[t]) / sqrt(1 - alpha_hat[t]) * predicted_noise) + sqrt(beta[t]) * noise
'''
logging.info(f"Sampling {n} new images....")
model.eval()
with torch.no_grad():
x = torch.randn((n, 3, self.img_size, self.img_size)).to(self.device)
for i in tqdm(reversed(range(1, self.noise_steps)), position=0):
t = (torch.ones(n) * i).long().to(self.device)
predicted_noise = model(x, t, labels)
# interpolate with unconditioned diffusion
if cfg_scale > 0:
uncond_predicted_noise = model(x, t, None)
# predicted_noise = predicted_noise * cfg_scale + uncond_predicted_noise * (1 - cfg_scale)
predicted_noise = torch.lerp(uncond_predicted_noise, predicted_noise, cfg_scale)
alpha = self.alpha[t][:, None, None, None]
alpha_hat = self.alpha_hat[t][:, None, None, None]
beta = self.beta[t][:, None, None, None]
if i > 1:
noise = torch.randn_like(x)
else:
noise = torch.zeros_like(x)
x = 1 / torch.sqrt(alpha) * (x - ((1 - alpha) / (torch.sqrt(1 - alpha_hat))) * predicted_noise) + torch.sqrt(beta) * noise
model.train()
x = (x.clamp(-1, 1) + 1) / 2
x = (x * 255).type(torch.uint8)
return x
def train(args):
'''
Training process:
each epoch, each batch, rand_time_step, compare the predicted noise with the real noise
'''
setup_logging(args.run_name)
device = args.device
dataloader = get_data(args)
model = UNet_conditional(num_classes=args.num_classes).to(device)
optimizer = optim.AdamW(model.parameters(), lr=args.lr)
mse = nn.MSELoss()
diffusion = Diffusion(img_size=args.image_size, device=device)
logger = SummaryWriter(os.path.join("runs", args.run_name))
l = len(dataloader)
ema = EMA(0.995)
ema_model = copy.deepcopy(model).eval().requires_grad_(False)
for epoch in range(args.epochs):
logging.info(f"Starting epoch {epoch}/{args.epochs}:")
pbar = tqdm(dataloader)
for i, (images, labels) in enumerate(pbar):
images = images.to(device)
labels = labels.to(device)
t = diffusion.sample_timesteps(images.shape[0]).to(device)
x_t, noise = diffusion.noise_images(images, t)
# 10% of the time, don't pass labels, unconditioned diffusion
if np.random.random() < 0.1:
labels = None
predicted_noise = model(x_t, t, labels)
loss = mse(noise, predicted_noise)
optimizer.zero_grad()
loss.backward()
optimizer.step()
ema.step_ema(ema_model, model)
pbar.set_postfix(MSE=loss.item())
logger.add_scalar("MSE", loss.item(), global_step=epoch * l + i)
if epoch % 10 == 0:
labels = torch.arange(10).long().to(device)
sampled_images = diffusion.sample(model, n=len(labels), labels=labels)
ema_sampled_images = diffusion.sample(ema_model, n=len(labels), labels=labels)
# plot_images(sampled_images)
save_images(sampled_images, os.path.join("results", args.run_name, f"{epoch}.jpg"), nrow=len(labels))
save_images(ema_sampled_images, os.path.join("results", args.run_name, f"{epoch}_ema.jpg"), nrow=len(labels))
torch.save(model.state_dict(), os.path.join("models", args.run_name, f"ckpt.pt"))
torch.save(ema_model.state_dict(), os.path.join("models", args.run_name, f"ema_ckpt.pt"))
torch.save(optimizer.state_dict(), os.path.join("models", args.run_name, f"optim.pt"))
展开/折叠
class UNet_conditional(nn.Module):
def __init__(self, c_in=3, c_out=3, time_dim=256, num_classes=None, device="cuda"):
super().__init__()
self.device = device
self.time_dim = time_dim
self.inc = DoubleConv(c_in, 64)
self.down1 = Down(64, 128)
self.sa1 = SelfAttention(128, 32)
self.down2 = Down(128, 256)
self.sa2 = SelfAttention(256, 16)
self.down3 = Down(256, 256)
self.sa3 = SelfAttention(256, 8)
self.bot1 = DoubleConv(256, 512)
self.bot2 = DoubleConv(512, 512)
self.bot3 = DoubleConv(512, 256)
self.up1 = Up(512, 128)
self.sa4 = SelfAttention(128, 16)
self.up2 = Up(256, 64)
self.sa5 = SelfAttention(64, 32)
self.up3 = Up(128, 64)
self.sa6 = SelfAttention(64, 64)
self.outc = nn.Conv2d(64, c_out, kernel_size=1)
if num_classes is not None:
self.label_emb = nn.Embedding(num_classes, time_dim)
def pos_encoding(self, t, channels):
inv_freq = 1.0 / (
10000
** (torch.arange(0, channels, 2, device=self.device).float() / channels)
)
pos_enc_a = torch.sin(t.repeat(1, channels // 2) * inv_freq)
pos_enc_b = torch.cos(t.repeat(1, channels // 2) * inv_freq)
pos_enc = torch.cat([pos_enc_a, pos_enc_b], dim=-1)
return pos_enc
def forward(self, x, t, y):
t = t.unsqueeze(-1).type(torch.float)
t = self.pos_encoding(t, self.time_dim)
if y is not None:
t += self.label_emb(y)
x1 = self.inc(x)
x2 = self.down1(x1, t)
x2 = self.sa1(x2)
x3 = self.down2(x2, t)
x3 = self.sa2(x3)
x4 = self.down3(x3, t)
x4 = self.sa3(x4)
x4 = self.bot1(x4)
x4 = self.bot2(x4)
x4 = self.bot3(x4)
x = self.up1(x4, x3, t)
x = self.sa4(x)
x = self.up2(x, x2, t)
x = self.sa5(x)
x = self.up3(x, x1, t)
x = self.sa6(x)
output = self.outc(x)
return output